How to Evaluate Forecasting Accuracy
- sujonodamario
- May 13, 2021
- 2 min read
Evaluating forecasting is important to know the performance of the forecasting itself and it is done by comparing the forecast values and actual values. Several methods are applicable for comparing two values and ideally, it depends on the decision maker’s loss function (costs, service level, etc.). However, general-purpose methods are worth considering when such information is unknown. One natural approach is observing the difference between actual and forecast and use the average to measure the performance.
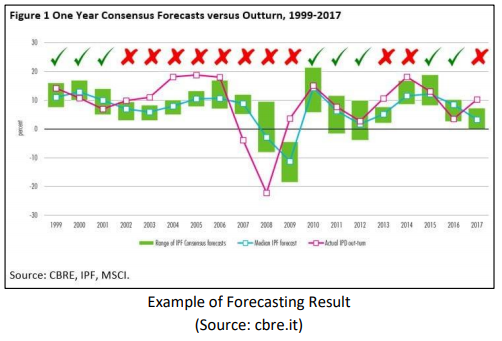
One example of such method is the Mean Error (ME). This measurement provides information regarding bias in a forecast: if the value is large (positive or negative), then the forecasts tend to be biased. Another variation of Mean Error is Mean Percentage Error (MPE): error is expressed as percentage which often more useful for strictly positive data. The formula for ME and MPE is shown below.
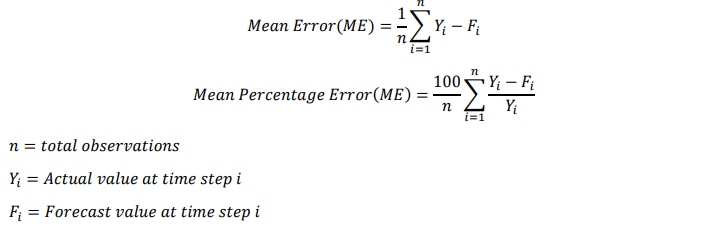
However, since ME and MPE include both negative and positive signs, it is possible that both positive and negative errors would cancel each other and as a result, the variability of the forecast is still unclear. Hence, Mean Absolute Error (MAE) and Root Mean Squared Error (RMSE) could break this limitation since making the value absolute and squaring the value would remove negative signs. RMSE is derived from Mean Squared Error (MSE) method which it provides greater weights to larger errors. It is more often used because MSE is measured in squared unit of measurement, thus, taking the square root of MSE would makes interpretation easier. The formula for MAE and RMSE is shown below.

All of these methods could be applied for one comparison and it provides more information of the performance itself. By evaluating the results from many different perspectives, decision-makers could use the combined information to prepare their next step accordingly.
Comments